So you’re a digital marketing specialist who works in the finance, retail, or automotive industries and tries to figure out how to scale new customer acquisitions and drive sales and LTV. You micro-segment your audiences, optimizing for lookalikes, but it still doesn’t bring you sound results.
We’ve been there and know how to cure it.
With predictive targeting, you can supercharge your conversions by 2-4x and see a sales increase of up to 45%. Let’s jump into the details right away.
Predictive targeting: what it is and why it matters
Predictive targeting is a data-driven approach to acquiring customers. It uses deep learning algorithms, predictive analytics, and customer intelligence to find high-value prospects that are more likely to convert.
What makes predictive targeting stand out is its ability to pinpoint the right audience with a high degree of accuracy. This type of targeting involves data about a consumer’s past behavior, demographic characteristics, and other relevant information to identify patterns and make predictions about their likely future actions.
For instance, Tomi.ai uses first-party behavioral data from your website and sales data from your CRM to calculate the purchase probability for every new visitor and feed this data to Facebook or Google via APIs.
Doing so helps ad platforms identify prospects that are more likely to buy right away and generate higher lifetime value.
Targeting is more than audiences.
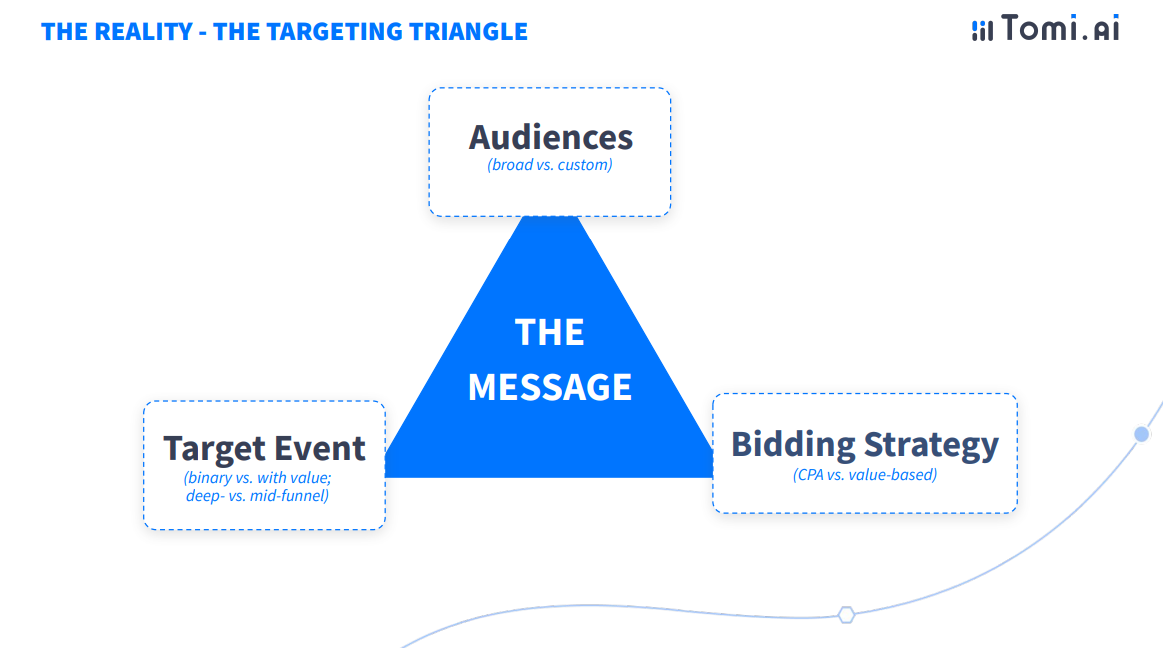
3 pillars of effective targeting.
There’s a common misconception in performance marketing that the more segmented your audiences are, the higher chances you will generate more sales-qualified leads at a lower cost. In reality, though, who sees your message (ads) at what time of their buyer’s journey are more affected by a target event and a bidding strategy.
In addition, we see more value in relying on broader audiences. Lastly, the ad message itself massively factors into engaging and converting advertising campaigns.
So babysitting your micro-segmented campaigns is not the best way to get the most out of Facebook and Google ads. What we suggest instead, let’s discuss in the next chapter.
4 elements of successful predictive targeting: audiences, bidding strategies, target events, and messaging
It’s important to understand the interdependent relationship between audiences, bidding strategies, and target events alongside the right messaging.
Audiences are the foundation of any targeting strategy. By breaking down the data you have at hand, you can create different audience segments that are more likely to convert. With a traditional approach, you would opt for narrow audiences, therefore, limiting Facebook or Google’s potential to find for you high-profitable customers within the broader audiences.
Bidding strategies allow you to allocate and optimize your budget in a way that gives you the most bang for your buck. Traditional performance marketing recommends bidding on CPA (cost per action) when predictive targeting favors a value-based approach, which helps build new larger high-performing lookalike audiences.
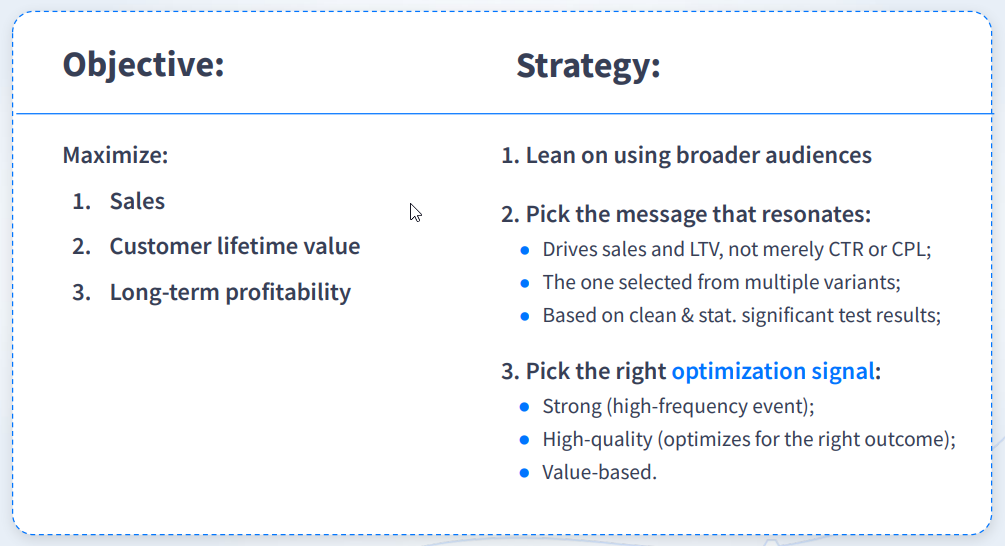
How to scale high-value customer acquisition with predictive targeting.
Target events are what you use to inform your bidding strategies. Traditional targeting implies target events like page visits, past purchases, sign-ups (for SaaS), or funded accounts (for finance). This approach might increase the number of leads but not necessarily translate into improved LTV since the sign-up event, for example, doesn’t guarantee conversions into paid customers.
In contrast, you can leverage predictive targeting and optimize for strong, high-quality events like highly-active customers within a certain period of time or with recurrent payments, etc.
Messaging should resonate with your target audience. It should be the one that not only generates clicks but drives high-profitable customers. How do you know which message will move the needle? Run a series of A/B tests to validate that message.
All that will help you maximize the impact of retargeting on marketing ROI and revenue. But how exactly? What to do next?
The optimization signal tradeoff and what to do about it
Optimization signals are the data points that are used to inform ad optimization decisions. The tradeoff is between relevance (targeting) and performance (bidding).
For example, if you’re targeting a very narrow audience, you can be sure that the people in your target audience are more likely to convert. But if the target audience is too narrow, you might not be able to generate enough conversions to justify your ad spend.
On the other hand, if you’re targeting a broader audience and using predictive analytics to optimize your bids, you might be able to generate more conversions. But the quality of those leads might not be as high as if you had been targeting a more specific audience.
However, if you optimize for target events, you can ensure that your bids are optimized for the right audience while also making sure that you’re targeting leads that are more likely to convert.
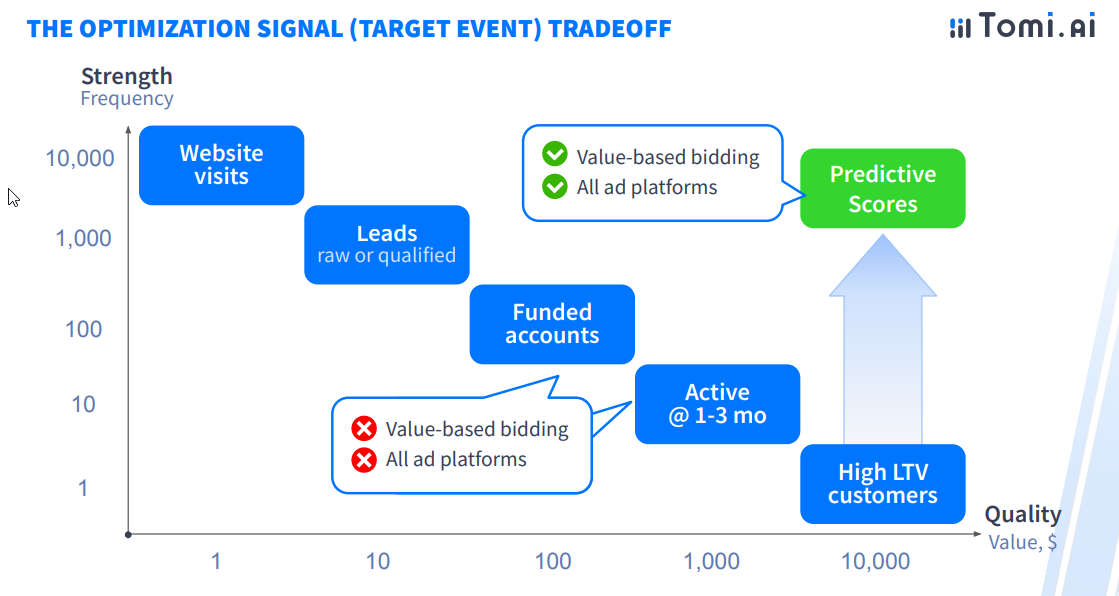
Target events for value-based bidding.
Let’s see how to apply this knowledge from a real-life example.
In the banking and insurance industries, there’s a clear trade-off — the stronger signal you have, like website visitors in terms of frequency, the less valuable this signal actually is. Will all those leads become funded accounts? Will they become high-LTV customers down the road? Likely not.
Here’s where predictive analytics comes into play. With that, you can build a probability distribution for your leads and website visits to understand, which visitor have a higher chance of converting into a high-value customer (e.g., the one who is approved for a credit card and then carries on the balance and pay you some interest).
Such predictive scores you can then utilize for value-based bidding to increase the acquisition of high-quality leads.
How to act on predictive insights?
So what to do next to scale high-value customer acquisition once you have the scoring model and collected customer behavioral insights? Let’s focus on two key strategies.
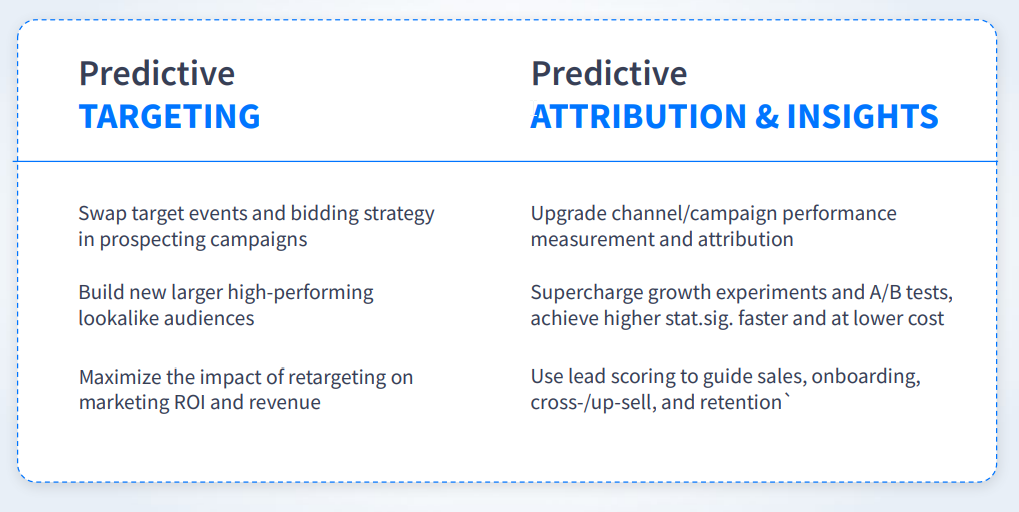
6 strategies of how to employ predictive targeting.
Swap target events in prospecting campaigns.
Instead of optimizing for leads or funded accounts, or sign-ups, regardless of their value, you can now optimize for predictions of the likelihood of the visitor becoming an active customer. For the cash loan industry, you can even optimize for the number of commissions these people are going to pay you.
Instantly, you have a tool to make Google and Facebook work harder to pinpoint the right audience for you.
Create larger high-performing lookalike audiences.
With predictive analytics, you can perform value-based segmentation to create larger lookalike and more precise audiences. As such, you can elicit people who are in the market right now seeking to get a new credit card and market your products to them with perfect timing.
Couple these two tactics and you’ll maximize your ROAS and ROI.
What results can you expect from predictive targeting?
We at Tomi.ai have successfully implemented the strategies discussed above for seven customers in the banking sector.
When we switch the target event from an actual funded account or a lead to a predictive event, we see about a 50% increase in approval rates on average. Next, the average increase in approved credit limit is about 25%, and the decrease in customer acquisition cost is 25-45%.
What’s more is that we managed to double the prospect-to-customer conversion rate.
However, how does it work in practice?
How predictive targeting works — the tech behind it
We’ve touched upon the basic technical aspects above, but here we want to get into the nitty-gritty.
Let’s consider Tomi.ai. First, you need to install the pixel on your website so that the machine can start collecting the behavioral data of your visitors. Then, connect it to your CRM to gather information about 300 sales that are happening during the period since the pixel is installed. Next, Tomi’s predictive model bridges sales intent and site visitors’ behavioral data and analyzes multiple data points to find trends and patterns common to high-value customers. This process takes four to six weeks
The next step is to design a predictive score (predicted purchase value) for every new visitor. The training of the model takes 1-2 weeks, but once it’s up, the calculation of the score for every new visitor takes less than 2 hours.
As such, we have the right data to send to Google, LinkedIn, or Facebook as custom audiences so platforms can better train their models to identify the right buyer signals and show your ads to those people.
Delegate ads optimization to ad platforms
All this may seem like magic, but it is more than real. If you want to start working with predictive analytics, begin to trust machine learning to predict the future.
Want to learn more about predictive targeting? Book a personal demo with Tomi.ai and unlock your growth!