Most advertisers use microsegmentation in an effort to improve their return on ad spend (ROAS). The reasoning seems sound: advertising randomly to everyone would be a waste of ad dollars. Targeting your ads to small audience segments that are most likely to buy from you should (and does) produce better results.
The problem with microsegmentation, however, is that by advertising only to the highest-converting sliver of your audience, you’re inadvertently ignoring the majority of your customer base.
Luckily, there’s a better way to hit your ROAS targets without sacrificing sales volume. Through newly available predictive optimization, you can still target your highest-performing audience segments without completely ignoring the rest.
Why advertisers love microsegmentation
In the world of online advertising, there are two traditional forms of audience segmentation:
#1 – Lookalike audiences – Targeting a lookalike audience means advertising to audience members that most closely resemble the people who have bought from you in the past. Ad networks compare demographics, interests, and behaviors to a list of customers you’ve provided.
For example, using Facebook lookalike audiences, you can upload a source audience of 100 to 5,000 customers. You can then use a percent range to tell Facebook how closely your new audience should match your source audience (e.g. target only the top 3%).
The Google Network has similar targeting settings, but calls these similar audiences and Customer Match.
#2 – Interest segments – The other segmentation option is to target audience members based on their personal attributes, interests, or demographics. This doesn’t require input about existing customers, but does require you to hand-select key audience
For example, Google audience targeting allows you to target audience members based:
-
- Affinity: Users’ habits and interests
- Demographics: Age, gender, education, income, marital status
- Life events: Users who are about to graduate, get married, or have a baby
- In-market: Users who have signaled a purchase intent in your industry
If you were selling baby clothes, for example, you could target expectant mothers with disposable income. In both cases, you take 100% of the people available to you, and then you limit that audience to a microsegment based on data or your theory of your ideal customer. Advertisers love this approach because these segments convert at higher rates and therefore produce better ROAS.
The problem with microsegmentation
The problem with microsegmentation is that it’s binary. You either advertise to someone because they meet your segmentation rules, or you don’t. It’s all or nothing.
By condensing your audience to only your best-possible customers, you miss out on the majority of your customers and therefore miss out on the highest-possible sales volume.
Therefore, a lot of people who would have been interested in buying your product—had they heard about it—will never hear about your product because they didn’t make it through Facebook’s interest segment or lookalike audience algorithm.
To put it in numbers: The top percentile of your audience could have a conversion rate of 48%, but your 20th percentile may still convert at 9%. Is it worth ignoring that 20th percentile customer completely? Definitely not.
How many customers are you missing out on with microsegmentation?
Microtargeting causes you to lose out on 50 to 90% of your total possible customer base.
How did we arrive at that number? Let’s walk through an example using real Tomi.ai data from a client in the financial services space.
For the purpose of this example, let’s bin the customer’s entire possible audience into 100 buckets, each with 1% of their total audience population. The buckets could be binned by attributes such as predicted lifetime value of a contact.
If the customer advertised randomly to all 100 buckets, the conversion rates for each bucket would be distributed like this, with some buckets converting more than others:
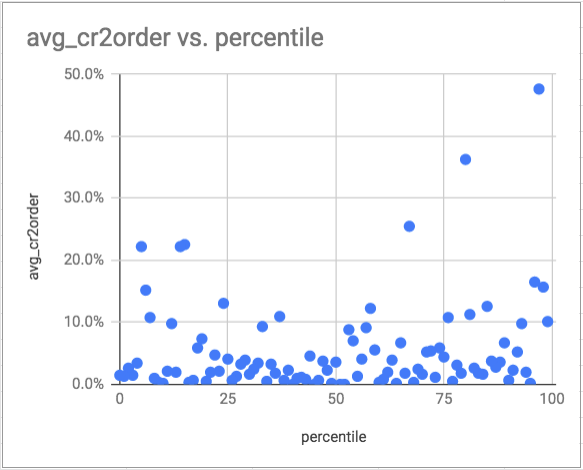
Each 1% bin is sorted randomly along the x-axis. Conversion rate is on the y-axis.
In this example, the best bin converts at 48%, the second-best bin converts around 36%, and so on. There are plenty of bins at 0% conversion rate. The average is around 5%.
If we sort these bins by conversion rate, they would look like this:
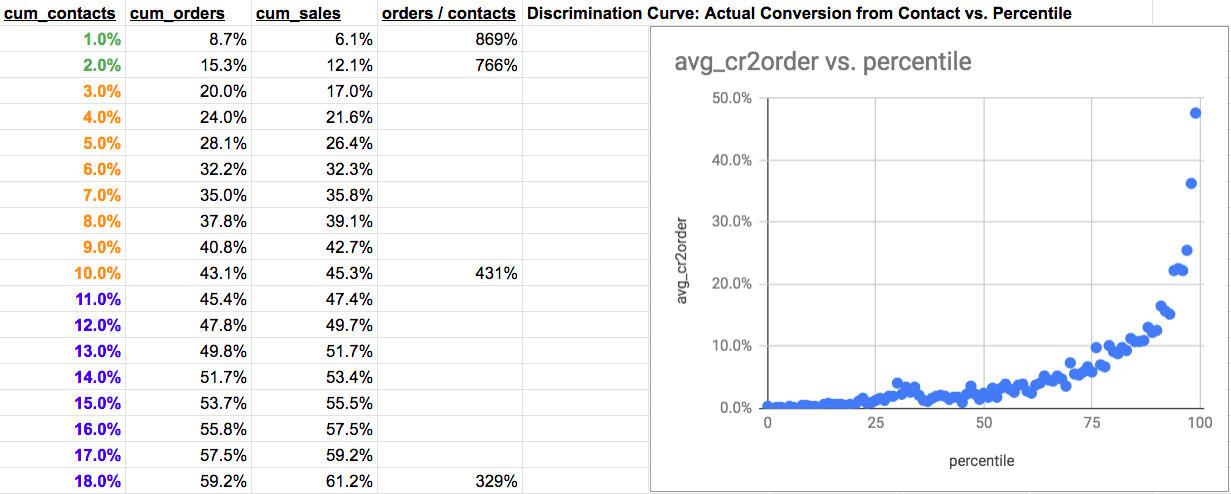
1% audience bins sorted by predicted conversion rate (least-to-highest converting)
Then, you can look at each 1% bin in terms of what cumulative percentage of the orders they produced:
Looking at the cumulative order data, we see that the top 1% of the audience provide 8.7% of the customer’s true conversions. This means we condensed the audience into a bucket that buys 8.7 times more than the average audience member.
Going down the chart, we see the cumulative top 2% of the audience provide 15% of the orders. Advertising to this segment would net you 7.6 times the ROI than advertising to a random bin.
The top 10% of the audience provides 43% of the orders—4.3 times the advertising ROI.
If the client were using microsegmentation, they would use these top-performing audience buckets to target their ads.
For example, they could create a lookalike audience on Facebook using the top 2% of the audience, or they could use the top 10% of the audience to try to create audience segments with the right interest categories. For example, they could target customers buying insurance, people who have cars, fans of BMW, etc.
What’s the problem here?
By targeting the top 10% of your audience who account for 43% of your orders, you’ve left out 90% of your population who account for 57% of your remaining potential orders.
How does predictive optimization solve this problem?
To deal with this problem, Tomi.ai takes a creative approach to targeting and optimization.
At Tomi.ai, we use predictive optimization. This means advertising to your entire possible audience, but only bidding at the dollar amount that satisfies your ROAS requirement based on the conversion data you’ve collected about that audience segment.
In contrast, microtargeting is binary. You separate the good audience members from bad audience members. You do this because the lower audience segment is too diluted to be profitable when you look at the average.
But if instead of microtargeting, you optimize your bid to the level of dilution for that segment—i.e. bid less for lower-converting audience segments—you can still advertise to your entire audience with a profitable margin.
How Tomi.ai’s predictive optimization works
To illustrate how predictive targeting and optimization works, let’s return to our example financial services client above.
- Website pixel – We start by installing a pixel on our client’s site to collect first-party visitor data. This allows us to track the on-site behavior of 100% of visitors over a period of time (e.g. one month).
- Customer data – We then combine the website behavior with the client’s CRM sales data over that time period to get a complete picture of customer behavior.
- Machine learning – Next, we use this customer data to train our machine learning models.
- Optimization signal – The model then outputs the probability of an individual visitor’s likelihood to convert.
- Smart bidding – Finally, the optimization signal data for 5 to 10% of highest engaged website visitors is fed into Google and Facebook’s smart bidding algorithm to bid the right dollar amounts for the right customers—without any targeting.
- Profit! – The client sees high ROI without cutting their audience.
The key to success here is better input data provided by the website pixel and customer CRM. By using this data to feed your predictive model, you can equip the smart bidding algorithms with a frequent as well as nn accurate optimization signal instead of resorting to segmentation.
In summary, bidding through predictive optimization means taking the stance that, “every segment is good for me, as long as I advertise to that segment for the right price.”
Because microsegmentation only addresses about 10% of your customer base who provide 30-50% of your sales, you can double or triple your sales with a more profitable optimization approach.
Why aren’t people already using predictive optimization?
If predictive optimization results in more sales, why are so many advertisers still stuck on microsegmentation?
The answer is that there have been two major changes in the market:
1. Ad platforms are becoming smarter
One reason why bidding optimization has become a reality is because ad platforms like Facebook and Google have been shifting more and more to auto-bidding and smart-bidding strategies.
The better the input signal for these algorithms, the more they can optimize for the right audience.
For example, e-commerce businesses used to advertise to lookalike audiences. But because e-commerce businesses can train the smart-bidding algorithms with thousands of conversions, the smart-bidding algorithms have more than enough information to optimize for value-based signals like conversions or revenue rather than relying on segmentation (outside of new vs. returning customers).
This is a bigger challenge for businesses with long sales cycles and fewer conversion signals. This is one problem we’re trying to solve with Tomi.ai—by integrating customer behavior and CRM sales data.
2. Ad platforms are more open to conversion API integrations
The other shift has come from the ad platforms’ willingness to provide server-to-server integrations. These platforms used to only allow targeting through segmentation and lookalike audiences.
For example, Facebook only allowed server-to-server data integrations as of 2020, which was later called Conversion API. Previously, these server-to-server conversion integrations were only available to their top hundred or so advertisers.
This allows providers like us to integrate client data directly into their ad campaigns, creating smarter bidding strategies.
Optimize for the right signals with Tomi.ai
It makes sense why advertisers rely on segmentation. It’s a simple way to target high-converting audience members. That said, it has the unintended side effect of cutting out the majority of your audience.
Wide targeting and smart bidding optimization is the better approach—provided you have the right and frequent enough signal to optimize for.
By tracking behavioral customer data with Tomi.ai, you can provide the advertising algorithms with a non-binary input that allows the ad platforms to optimize for the right signal: the conversion likelihood value for the highest engaged potential customers.
The end result: more sales without sacrificing your ROAS.
To learn more about how Tomi.ai can win you more paying customers, contact us today.